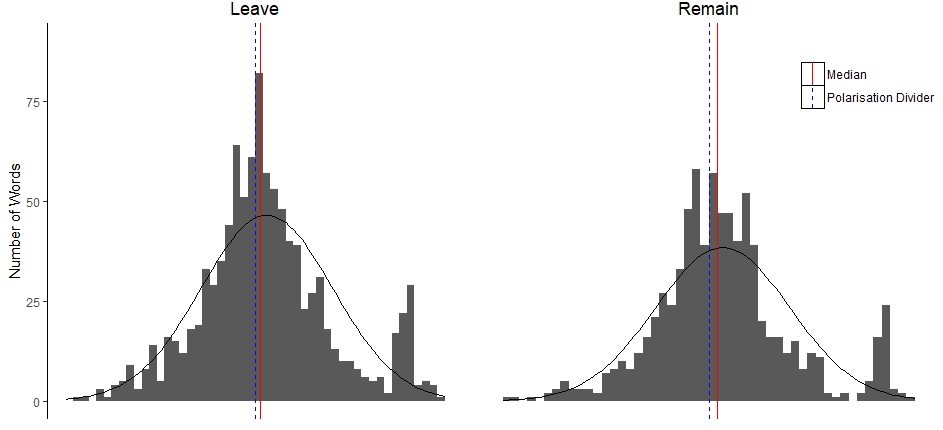
Tweeting Brexit
Brexit is an event with some strong opinions. I’ve used my Twitter scraper to find over 230,000 Tweets from the UK that talk about “Brexit”.
When I began writing this post I expected to see obvious clusters of words around leave vs remain, deal or no deal, labour vs conservative but the world is more complicated than this.
The message I’ve seen is regardless of position no one’s happy with how it’s being run. We see people that want an election, or another referendum or they want an election but no one is worth voting for while some just want to get on with it and leave regardless.
I’ve constructed this interpretation with a blend of statistical, visual and subjective analysis of Tweets. I have tried to present data that lets you draw your own conclusions but invetiably the interpretation I have drawn will show through. One Tweet in my set sums the landscape up pretty well:
I think #c4Brexit did a good job on balancing the panel. They had a (fairly) strong representative from each of the four main Brexit options that seem to realistic at the moment:
— Simon Banfield (@SimonABanfield) December 10, 2018
Deal, No Deal, General Election, People's Vote
Right now it isn't just about Leave or Remain.
Let’s start with a word cloud of the top 130 words used when talking about Brexit. I’ve removed words that don’t say anything useful by themselves - words like “but”, “if” and “or” (the text analysis pros call these ‘stop words’). I’ve also stripped out most punctuation, keeping some of the useful symbols we see in Tweets like “#” and “@”. I’ve not done anything fancy to the words themselves, so “vote” is a different word than “voted”, “voter” and “#vote”.
The top words are “will”, “deal”, “vote” and “people”. I’m suprised that “leave” and “remain” aren’t more prominent, this may be because I’m getting Tweets as the scheduled leave day approaches so the leave vs remain discussion must not be the main item of discussion.
The Day Approaches
The referendum for the UK to decide if it wants to stay in the EU took place back in June 2016. Following a 52% result to leave, the government at the time promised that the UK would leave the EU by 29th March 2019. I’ve been gathering Tweets on Brexit in an ad-hoc fashion since the 9th December 2018 and in Jan 2019 I updated my Twitter scraper so that I get every Tweet made about Brexit from the UK that I can using the search API. In the chart below I show you how many Tweets I’ve received each day with a few key events highlighted.
I received a large number of Tweets around the vote of no confidence on 12 Dec 2018, the vote on the deal, vote on delay of brexit, when 7 Labour MPs quit, around the article 50 extension and the indicative votes. These are all events discussing the business of Brexit, rather than people voicing opinion on whether they want to leave or remain as I hoped to see. I’ve broken this down by the top words (along with “leave” and “remain”), you can click the chart to make it interactive.
We see how on most days “will” is the most popular word but this changes during parliamentary votes. The use of words during the votes become more apparant when we look at proportional use amongst these words. You can also make this chart interactive by clicking it.
We see that the ratios between words stay roughly the same except during votes (like the 15th Jan during the vote on the deal) when the use of “vote” spikes - shocker! “Leave” and “remain” are fairly consistent throughout, with “leave” talked about more. This suggests much of the discussion is about leave and the democratic process around it. This is further supported when looking at the top 50 most popular words used along with leave and remain and how they correlate.
There isn’t any particularly strong correlation between leave or remain and other words, but voted, voters and vote stand out with leave.
Will. Deal. People. Vote.
We can imagine “will”, “deal”, “people” and “vote” (the most popular words in my set of Tweets) appearing together in phrases like “will of the people”, “the people need a vote”, “vote on the deal”. I’ve used hierachical clustering to look for clusters arond the most common words and plotted them on a dendrogram. I give a simple description of how to read a dendrogram towards the end of exploring rude britannia (it contains very rude language!) but briefly, it finds the strongest relationship between words and connects them by a line. If the strongest relationship is with a word that’s already paired up, the words get connected in a group.
The chart uses the 100 most common words that are Tweeted alongside at least one of “will”, “deal”, “people” or “vote”. (You can click the chart to rotate it).
The clusters have shifted as I’ve gathered more Tweets and it’s been quite hard to get meaning from the dendrogram but “mays deal”, “peoples vote” and “voted leave” (a phrase which groups up with remain!) have been quite consistent. Everything else falls into weak groups but we do see “will happen” and “british people” forming groups. These clusters allude to ideas of democracy: either asking for a “peoples vote” on “mays deal” or going with the “british people” who “voted leave”. I’ve selected a sample of Tweets from a few of these groups.
3 Brexit options
— Femi (@Femi_Sorry) January 16, 2019
▪️No Deal: The Brexit revolt was caused by politicians crapping on people's living standards - Makes it much worse
▪️Deal: Both sides blame the other for 'Bad Brexit'
▪️People's Vote: Those in positions of influence who've got the message push for POSITIVE change
Theresa Mays Brexit deal is dead in the water.
— Julie Harrington 🌹#NoPVYesGE #GTTO 🌹 (@celtjules66) January 2, 2019
She can’t run away from it for much longer.
It’s past time that we had a GE to get rid of this useless government once and for all.#JC4PM2019 🌹🌹🌹
Not a single British voter voted for a No-Deal Brexit. Theresa May can take a crash out Brexit off the table tonight. She refused to do so because she wants to play Russian Roulette, Her Deal v No Deal, with the British People. We in Labour refuse to play such a reckless game
— Tory Fibs (@ToryFibs) January 16, 2019
Theresa May should be on her knees begging fir forgiveness off the British People for her treacherous behaviour. She has wasted 2yrs of negotiations and purposely not allowed Brexit Secretaries do their jobs. She has done her best as a remainer PM to scupper Brexit. Disgraceful✌
— ❌PAUL MERRITT#IAMTOMMY🇬🇧# BREXIT🇬🇧#UKIP🇬🇧GB (@PaulMer45790900) January 14, 2019
So far the clearest thing we’ve seen is the word “leave” is used more than “remain” and that there is no obvious voice as to how this should happen. I suggest this is because we close to the leave date and there is no consensus on what to do and that eveyone is a bit annoyed with the whole process.
I can look at lave vs remain a bit more to try and ascertain a common opinion.
Leave vs. Remain
I was hoping to see nice clusters of correlated words around leave and remain which would give an insight into peoples opinions about Brexit. Instead we see how noisy the Brexit landscape is.
To see what people are saying about leave or remain, I test words used in conjunction with either side. For example, if a test word is used with the target words (leave and remain) in equal amounts (it can be in seperate Tweets) then the word is polarised equally between leave and remain (0.5). If a word is only ever used with leave, it is fully polarised to leave (1) and if it is only ever used with remain, the word is fully polarised to remain (0).
Using this we can hopefully see what people are saying about the major sides of Brexit, so if we were to see “don’t” heavily polarised towards leave, we might conclude that the people of Twitter don’t want to leave. You can see the results in my plot of the polarisation of words that are used more than 50 times.
We can look for unusual groups of words by counting where they sit in a histogram and fitting a normal distribution over the top. I only count a word once, so if it’s a popular word it doesn’t make the bucket bigger. I’ve highlighted the buckets that contain the words leave and remain.
I could possibly fit a better distribution over the top but it’s good enough as a guide to the eye. If the two target words did not form a relationship (perhaps because they’re both from different languages or certain sets of words don’t make sense together), we might expect that the histogram doesn’t follow a normal distribution, maybe with a big gap in the middle and all the words grouped at the edges close to the target words.
We see how words are generally used more with leave, which makes sense because leave is talked about 68% more than remain. I can account for this by weighting the counts of the test words with how often the target word is used i.e. before seeing how polarisied a test word is towards leave or remain, I weight its use with how often leave and remain are used.
Now that the use of words alongside leave and remain is normalised, we might assume that words are used randomly with leave and remain. The normal distribution fitted over the top shows what may be outliers (words that don’t follow the random use assumption) at the tails, notably bins with “remain” and “#wtobrexit”. Having “remain” outside the tail might be a sign that in the discussions, “remain” is used less than might be expected in the leave vs remain debate and could indicate people are settled on leaving.
I’ve made a wordcloud of the terms used to build the normalised histogram and coloured any word that is used more with remain in blue, and leave in orange.
We see “voted” and “people” with “leave” but also “vote” and “referendum” with “remain” so as before - quite chaotic!
Party Polarisation
I can use this idea of testing words against a target to see the polarisation of words between political parties and leave or remain. The left side of the histogram is the political party and the right side is leave/remain.
We see that the parties form a relationship with leave and remain but there are spikes of words in the tails, indicating words that are used more with leave/remain (or less with labour/tory) than we might expect when looking at these pairs of target words. This is particularly noticeable with the tory party.
This word cloud shows words in the spike on the right of the tory remain chart. The size shows how often the words are used, I’ve excluded words that aren’t used often.
We get “conservatives” as part of this group, probably because people rarely use “tory” and “conservatives” in the same Tweet. We also see a host of words that are particular to the Brexit debate like “wto”, “border”, and “#nodeal”, suggesting some words that might transcend party politics. This could be an avenue to see meaning in the noise of Brexit Tweets but I’ve already spent enough time writing this post.
Close
I’ve tried to see what people really think about Brexit but found the landscape incredibly chaotic. I’ve seen that leave is talked about more than remain, and this is probably because we are currently going through the long process to leave. We also saw nothing correlated particularly strongly with leave or remain, but there were weak links of “vote leave”. I found the most common words were “will”, “deal”, “people” and “vote” and clustering words around these showed some of the key phrases we’ve been hearing recently.
I think the chaos is because we are seeing democracy in action around a very complex topic. Brexit evokes some strong opinions and has many nuances that will inevitably require much compromise. However, many strong opinions and compromise don’t sit well together. No one person or group gets there asolute way and no clear voice is heard.
Thank you for reading.